Introduction
Diabetic retinopathy is the leading cause of blindness and the most common diabetic eye disease. There are approximately 93 million people with diabetic retinopathy worldwide. While the exact mechanism by which diabetes causes retinopathy is unclear, several clinical features are used to accurately grade and classify diabetic retinopathy. The following features can commonly be identified in fundus images:
- Microaneurysms: Appearance is small, red dots in the superficial retinal layers
- Retinal hemorrhages: Appear similar to microaneurysms if they are small
- Hard exudates: Appear as small white or yellowish white deposits with sharp edges
- Soft exudates (cotton-wool spots): Frequently bordered by microaneurysms and vascular hyperpermeability.
- Macular edema: Macular edema often causes mild opacification of the involved retina which appears slightly milky in comparison to the surrounding retina. Leading cause of visual impairment in patients with diabetes.
Adapted from COMS Grading Scheme
It is apparent that many of these features can't easily be quantified and their severity is also determined by the presence and relative positions of other clinical features. While image processing algorithms could be developed for each individual clinical feature, the correlations between the clinical features and their spatial positions cannot easily be accounted for by stacking individual image processing algorithms. Given the complexity of this image analysis task, we used the SemanticMD Compute Cloud to train a deep learning network for classifying images with diabetic retinopathy.
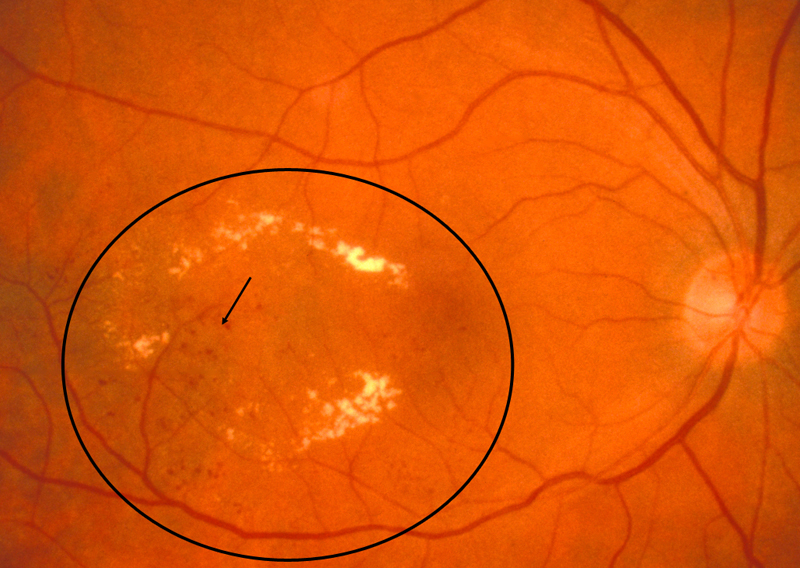
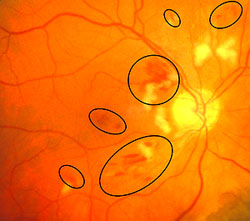
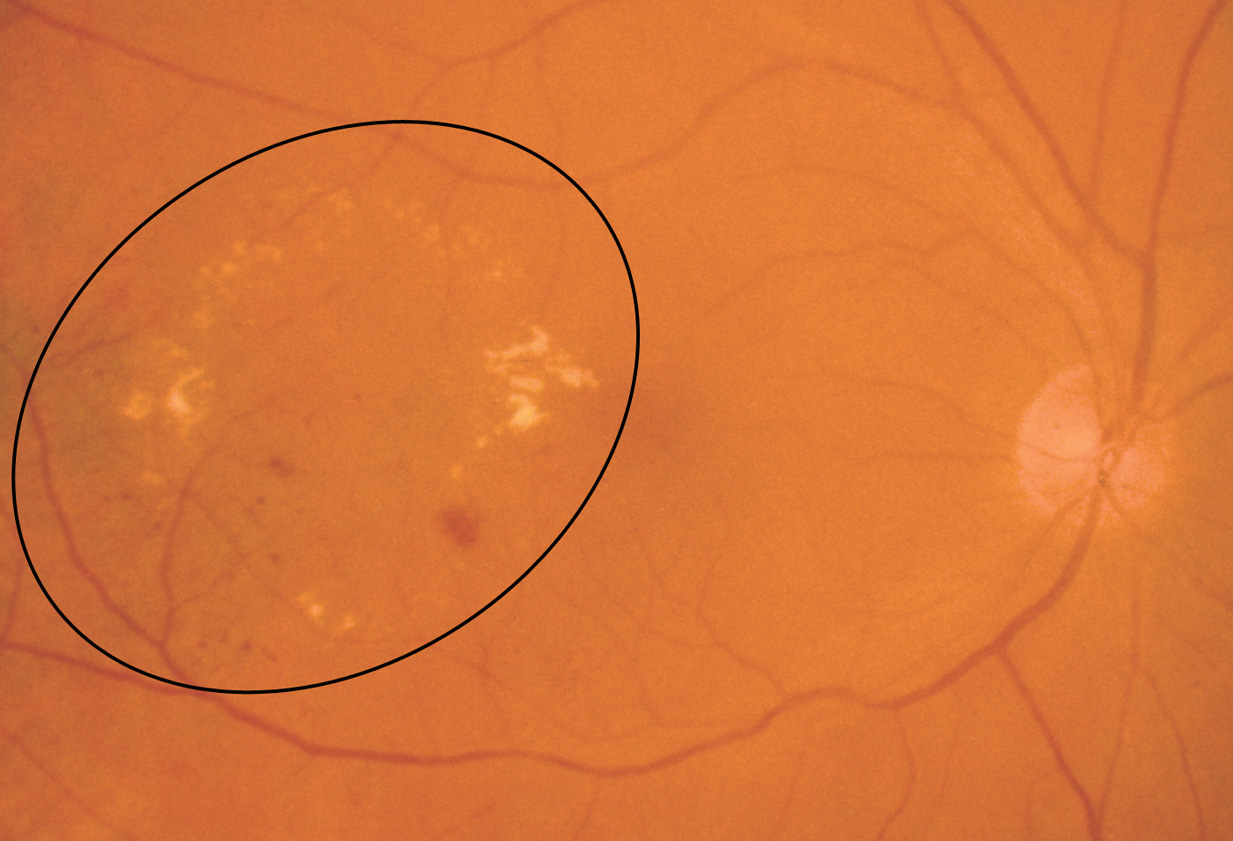
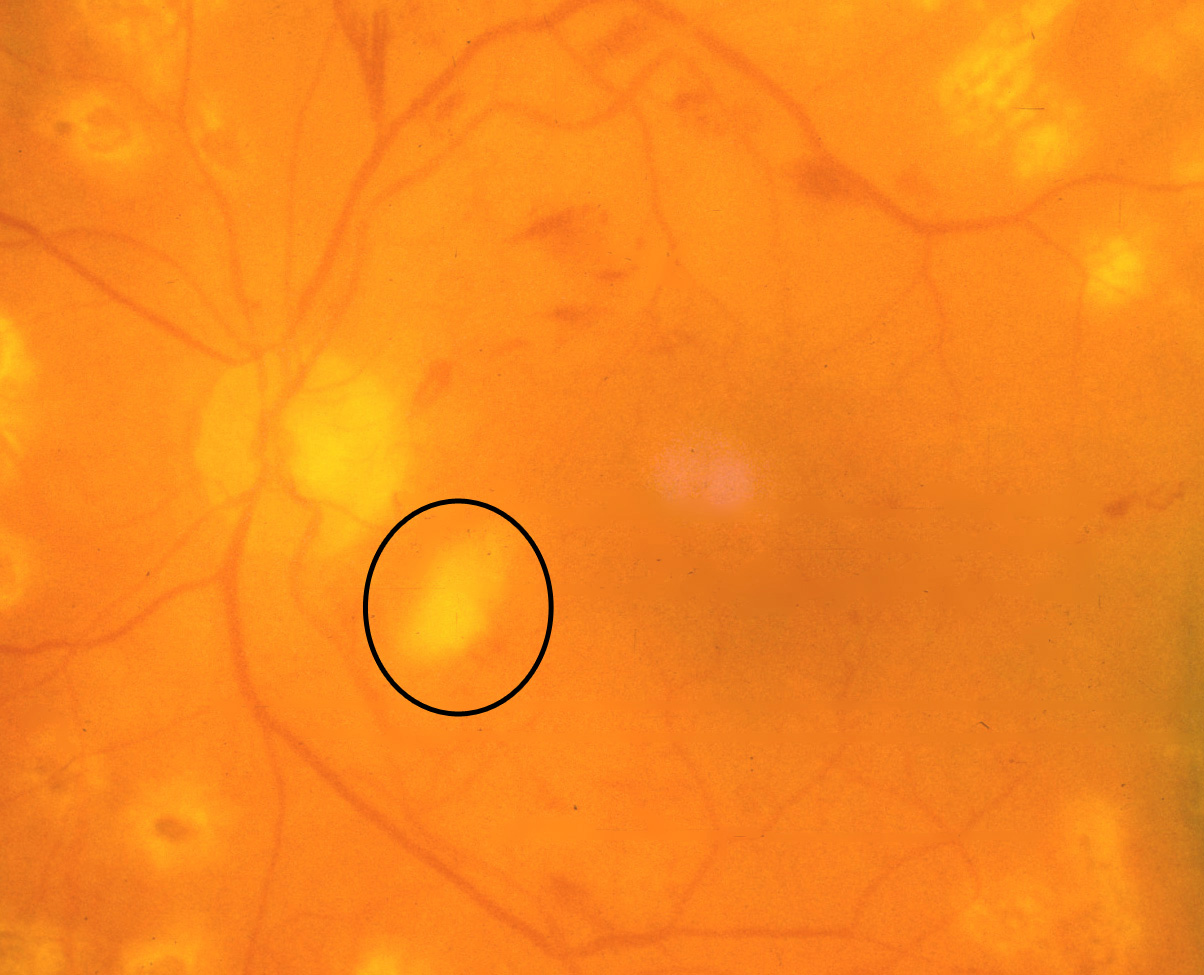
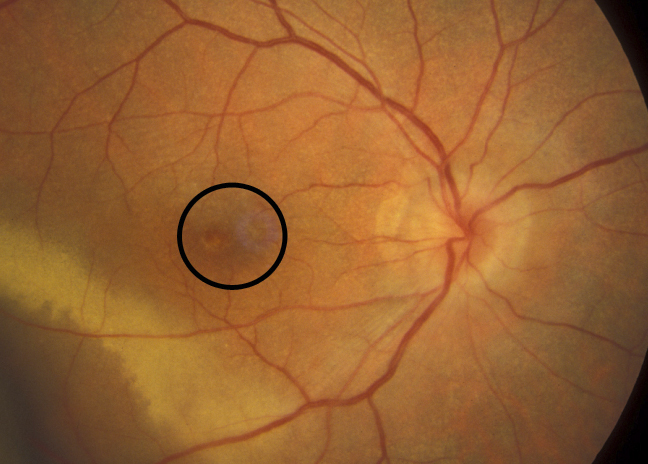
Platform Overview
The first step was to use SemanticMD Annotate Cloud to manage and annotate fundus images using a web-based platform. This enabled a team of trained imaging technicians to quickly process hundreds of fundus images within days. Once the data was organized and categorized, SemanticMD Compute Cloud was used to ingest the annotated fundus images and produce a web demo of a production-ready deep learning model. We host state-of-the-art deep learning libraries such as Caffe and Keras (Theano wrapper) so our models are continually up-to-date with the latest research.
Contact us below to find out how you can integrate SemanticMD Cloud directly into your imaging archive or medical device.
Deep Learning Pipeline
Pre-processing
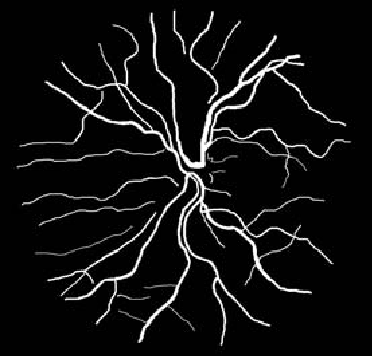
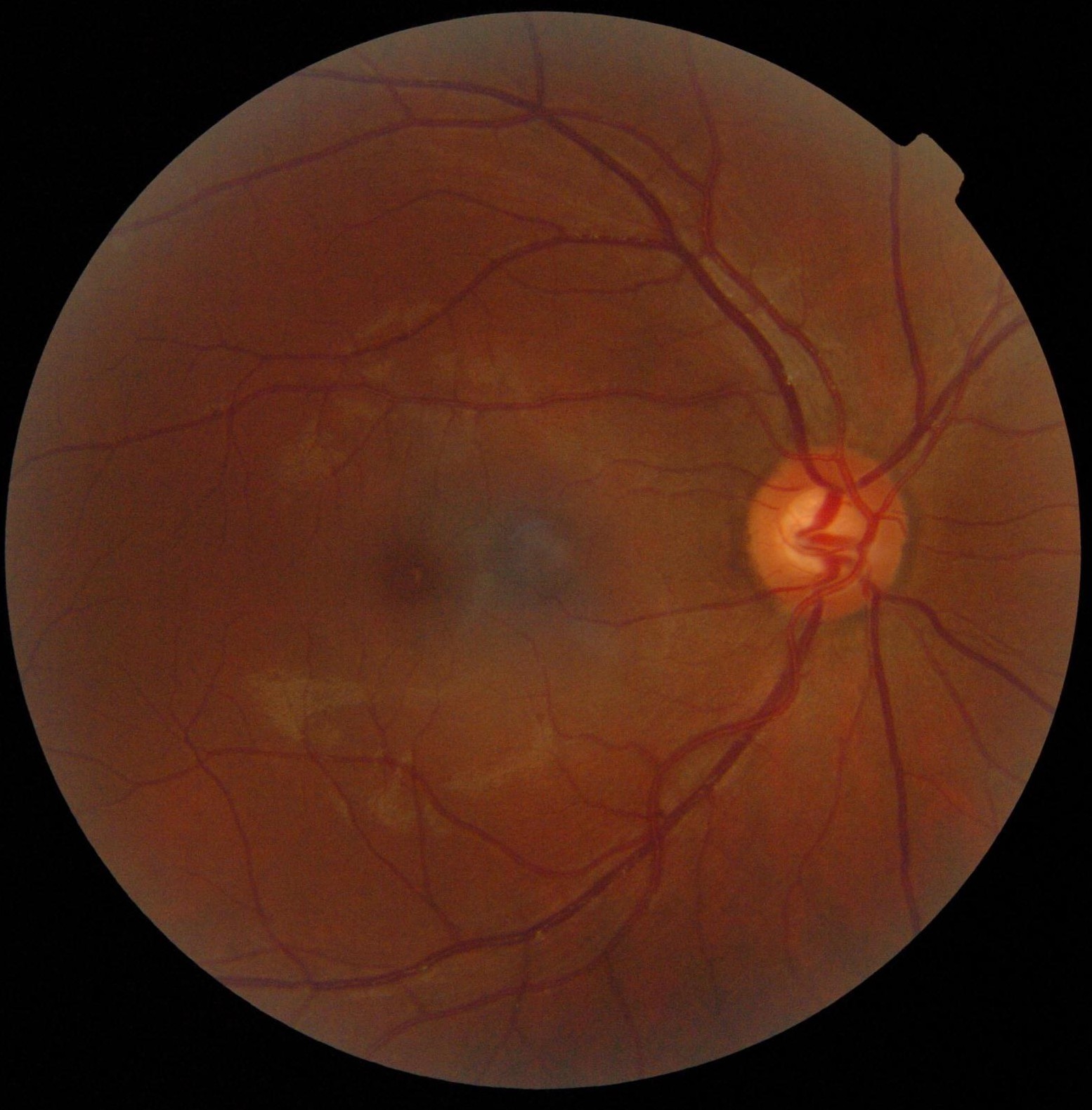
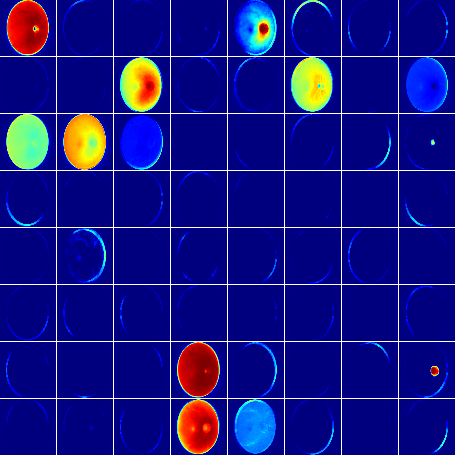
Blood vessel segmentation
Data augmentation:
- Color/contrast
- Flipping images
- Affine transformations
- Gaussian noise
- Local elastic deformations
Fundus image normalization
Model Training
Network architecture:
- Custom GoogLeNet
- Stacked input channels
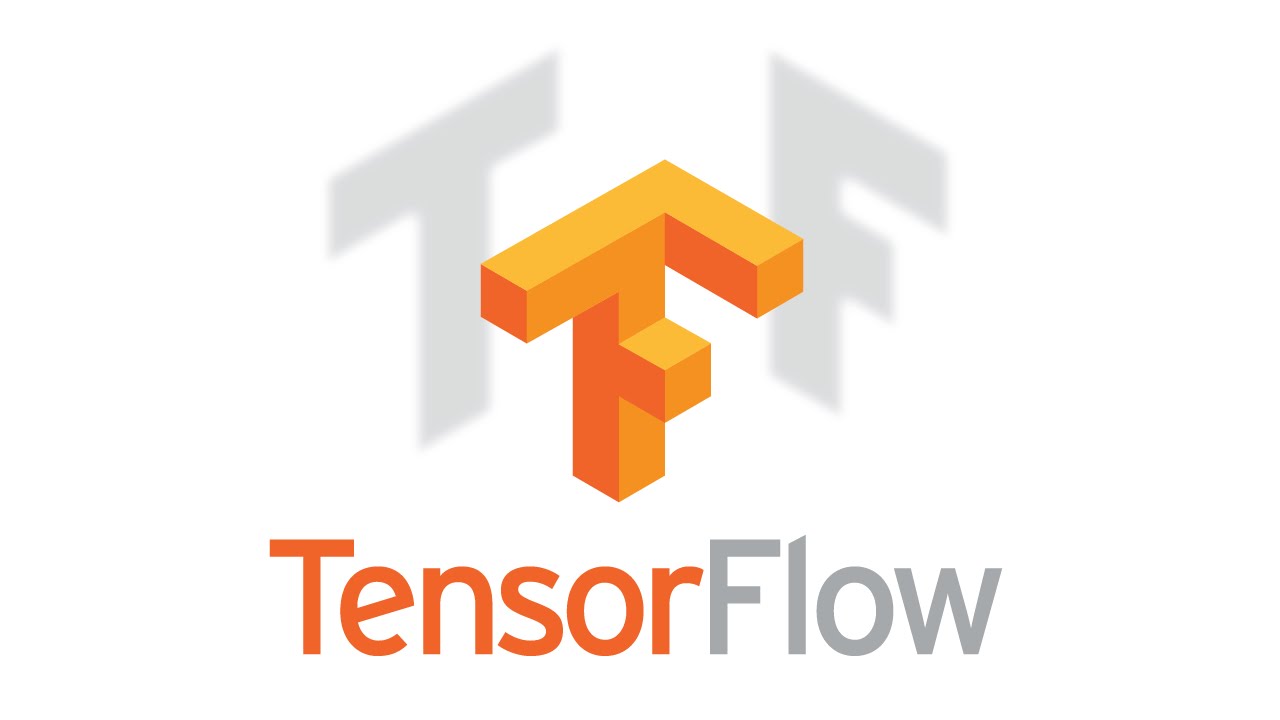
Deep learning libraries
Classification
We provide integration services that enable your customers to use SemanticMD Cloud models directly from your platform.
Take a look at our Developer Hub for more ways to use and integrate SemanticMD Cloud into your workflow.
30+
10+
7
million images
third party apps
minutes to join
"SemanticMD Cloud uses the latest advancements in deep learning and web technologies to accelerate R&D and develop products - fast."
